In the fast-evolving landscape of autonomous vehicles and ADAS, enhancing visibility through imaging and vision systems remains a critical challenge. Engineers in the field are well aware that the precision and reliability of these systems are paramount to ensuring safety and functionality. Current challenges include dealing with variability in sensor performance, maintaining image quality in low-light and adverse weather conditions, and the limitations of traditional metrics like SNR and dynamic range.
In this article, leading experts from INRiM, onsemi, Bosch, Imatest, Sheba Microsystems, Hyundai MOBIS and indier Semiconductor will give their insights through key questions on these challenges – including metrological techniques, camera performance indicators and V2X technology.
At AutoSens Europe 2024, industry experts will explore the latest innovations aimed at overcoming these hurdles, focusing on advanced sensing technologies, new performance metrics, and integrated approaches that promise to push the boundaries of what ADAS and autonomous systems can achieve. Check out the full agenda here.
To introduce the topic, we asked Paola Iacomussi, Senior Researcher at the Applied Metrology and Engineering Dept, INRiM: Can you discuss any innovative metrological techniques or methodologies that have recently been developed at INRIM (or with Bellaroma/CIE) for enhancing ADAS systems?
Paola: Bellaroma group is working together with the CIE Research Forum RF 06 Toward a new reference observe Non-Biological for defining a new reference to increase road safety. Cameras and sensors (e.g., lidar) used in ADAS are crucial for a vehicle to sense and perceive road surroundings and act to increase driving safety. Camera systems have been developed over many decades with the human visual system as a reference, both as a technical basis (e.g., the choice of colour filter arrays to mimic human color recognition) as well as the final application, providing an image to the driver. Thus, driving is no longer a human-only visual task and this reliance on the human visual system limits the development and performance of ADAS functionality, as ADAS sensors have distinctly different capabilities than the human visual system.
Bellaroma and CIE are working together to move beyond the photometric measurements (CIE standard photometric observer) under reference conditions (CIE geometrical reference conditions), as stated in existing standards and CIE reference documents about road environment. By abandoning the preconception of photometry, it is conceivable to convey the semantic meaning of road signs (i.e. the meaning of a yellow road marking) by a radiometric signature easily identifiable by ADAS systems. But obviously, this is predicated on the knowledge of radiometric characteristics of road environment (materials and light / radiometric sources) and of metrological performances of ADAS systems.
The first steps Bellaroma is pursuing is to characterize radiometric signature of road materials (like road marking) on larger than visible range and to build digital twin of sensors by doing metrological characterizations of sensors.
The shift in vehicle control systems from a biological photometric response to a non-biological one would provide considerable opportunity for safer systems. It is also an opportunity to combine the best of biological and non-biological observation of road environment and signalling for efficient and effective safety in vehicular activities and incidents.
DON’T MISS PAOLA’S SESSION AT AUTOSENS EUROPE
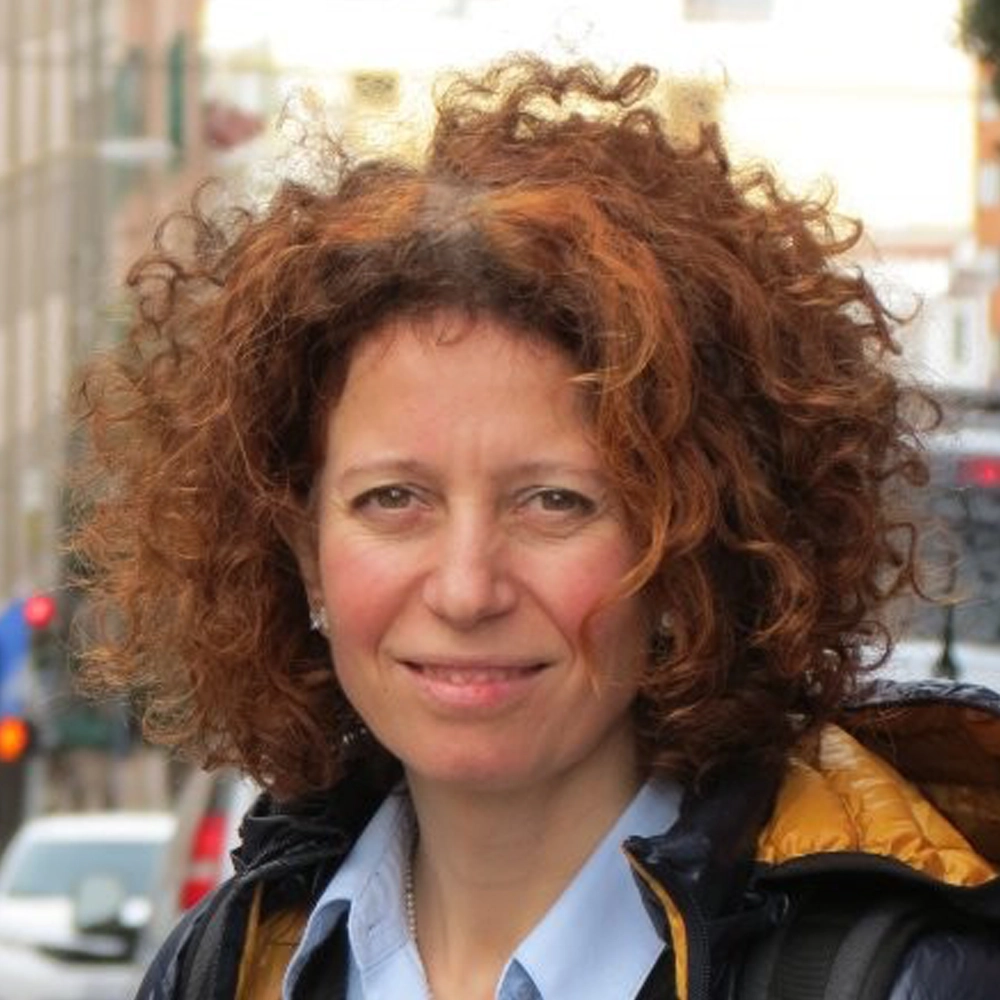
Improving road safety by optimizing road requirements for ADAS sensing
Paola Iacomussi
Senior Researcher, Applied Metrology Dept,
INRiM
To dive into this topic further, we wanted to hear from our experts on what the primary indicators used are to evaluate the performance of camera systems in automotive applications, and how these indicators impact the overall effectiveness of ADAS and autonomous driving systems.
Read on for insights from Norman Koren, Founder and CTO of Imatest; Radhika Arora, Sr Director – Product Management at onsemi and Dr. Peter Vastag , Engineering Camera Technology 2 (XC-AC/ECT2) at Robert Bosch GmbH.
Norman: To predict ADAS or autonomous driving system performance, we must go beyond elementary, direct measurements such as sharpness, noise, and Signal-to-Noise Ratio (SNR), and use a new set of metrics, derived from the direct measurements, but based on information theory. The new information metrics include information capacity, ideal observer SNR (SNRi)— an indicator of how well an object can be detected, and edge location standard deviation (σ)— an indicator of how accurately it can be located. The key information metrics— SNRi and Edge location σ— can be optimized by including a matched filter in the Image Signal Processing (ISP).
The new metrics can be measured most conveniently with slanted edges, using a new robust technique for measuring noise in the preseSnce of test images, and they can also be measured with Siemens star and Dead leaves test patterns. Because the information metrics are so new, there is much to be learned. Their predictive power needs to be verified, most likely using a labeled image data set with degraded and filtered images to correlate them with classic performance metrics such as mAP and IoU. I would guess the new metrics could drive a modest performance improvement, perhaps ten or twenty percent, which is not insignificant in the large and cost-sensitive automotive industry.
Radhika: The performance of image sensors in automotive applications is evaluated using several key indicators.
Noise is one of the biggest parameters impacting sensor performance and in turn ADAS performance. Noise can be in the form of FPN (Fixed pattern noise), dark current noise, thermal noise, etc.
Signal-to-noise ratio (SNR) is the average ratio of signal power to the noise power. Average SNR is less important than consistent and high SNR across all parts of an image, especially when it comes to varying light levels (shadows, bright sunshine, or a dark night).
The performance can also be adversely affected by wide variations in ambient lighting conditions (example, bright sunlight incident at a low angle of elevation or reflecting from a wet road surface) and other light sources such as the headlights of oncoming vehicles. HDR (high dynamic range) is a good indicator to mitigate these corner cases.
Temperature is always a challenge for image sensors and can significantly degrade image quality and performance. This is particularly true in automotive applications where sensors run at elevated junction temperatures of 80°C or higher for more than 80% of their lifetime—due to being placed in direct sunlight and designed in small enclosed spaces with other electronics that generate heat during operation. It’s imperative that key metrics for imagers demonstrate consistency in performance for more predictability.
Peter: The performance of imagers in automotive application is often broken down to few global performance indicators as dynamic range or signal-to-noise-ratio (SNR). While these indicators give a first impression about the typical average performance of an imager, they often tell little about expectable part-to-part variations or fluctuations between different pixels within one imager. At the end, the effectiveness of ADAS and autonomous driving systems will depend on the performance of the actual physical sample of the imager contained within the camera set mounted into the vehicle. Therefore, algorithms and their training and release need to rely on the validity of the underlying image data which should reflect variations in imager performance to ensure a proper and safe functionality for each and every vehicle. As wafers and wafer lots contain tens of thousands of imagers, each with individual performance, reflecting all of this variation in vehicle image data recorded with physical samples is not feasible.
Furthermore, global performance indicators as SNR are composed from different figures (as signal and noise) and not directly monitored in state-of-the-art screening processes for imager manufacturing. Yet, there are established models on how the transformation of incoming light to an image signal is done for CMOS imagers. These sensor models consider a set of “classical” parameters related to the pixel (and sensor) performance, e.g. dark current, and allow for reproduction of parameters as SNR. Moreover, these parameters are usually closer to actual imager failure modes considered during screening. Characterizing and monitoring these parameters on a standardized way could give a reliable prediction of expectable image quality also for corner cases which cannot be reflected in actual image data in a simple way.
CATCH NORMAN’S TUTORIAL AT AUTOSENS EUROPE WITH A FULL PASS
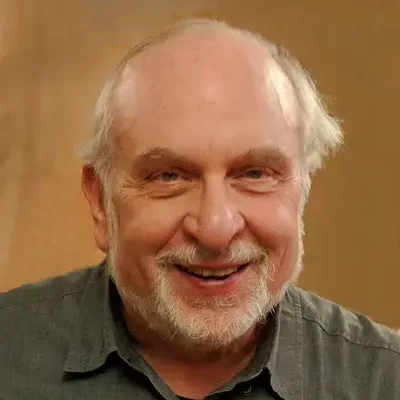
Information Metrics for Performance and Optimization of Machine Vision Systems
Norman Koren
Founder & CTO
Imatest
We’ve explored the key indicators, but how are advancements in camera technology addressing the challenges of low-light conditions and adverse weather specifically? We asked industry experts Marty Agan, Director of Automotive Marketing (Vision BU) at indie Semiconductor and Dr. Faez Ba-Tis, CEO and Co-founder at Sheba Microsystems Inc. to share any recent breakthroughs or promising approaches that significantly enhance camera performance in these difficult environments.
Marty: Recent advances in automotive camera technology are leading to improved low-light performance while maintaining color fidelity, which has benefits for both computer vision-based Advanced Driver Assistance Systems (ADAS) and human-viewing applications. A new breakthrough in image signal processing (ISP) for image sensors with alternative color filter arrays (CFA) is now making this possible. Traditional Bayer CFA’s with red, green, and blue filters (RGGB) lose about 2/3’s of photons incident on the sensor. Low-light sensitivity is much higher for alternative CFA’s that replace some color filter elements with more transmissive elements such as red-clear-clear-blue (RCCB) or RCCG. Such alternative CFA’s have not previously been widely deployed with existing ISP technology due to unacceptable noise and artifacts that can ultimately impact computer vision algorithms (for example, red lights are rendered orange or white). Improved automotive vision sensing in low light enabled by the latest-generation of ISP offers tremendous benefits to vulnerable road users (such as pedestrians and cyclists) and will enable higher performance next-generation smart cameras, e-Mirrors, surround view systems, and In-Cabin occupant and driver monitoring systems (OMS/DMS).
Dr. Faez: Automotive cameras often face challenges in maintaining image quality and focus stability in low light, adverse weather, and temperature fluctuation conditions. And, as the automotive sensor market continues to trend towards higher resolution sensors, this problem becomes increasingly pronounced due to the high resolution sensors’ smaller pixel size. This is because smaller pixels actually require even more precise alignment of the optics to ensure that light is accurately focused on each pixel, and any misalignment due to thermal expansion or contraction can lead to blurring or distortion. Of course, this is problematic for any camera but it is an especially huge issue for automotive cameras due to the extended temperature range.
This is exactly what Sheba’s solving. Our proprietary micro-scale electrostatic actuators uniquely move the lightweight sensor along the optical axis, instead of moving the lenses, which significantly enhances camera performance even in the world’s most extreme environments. Sheba actuators are thermally stable, and maintain consistent performance from -40 to 150 degrees Celsius, and have passed all drop, thermal shock, thermal cycling, vibration, mechanical shock, tumble, and microdrop reliability tests.
Ultimately, the adoption of higher resolution sensors in automotive will unlock powerful advantages, such as ultra-precise object detection (pedestrians, animals, road signs) and digital zooming. Especially with today’s advancements in autonomous vehicles, making sure these cameras work to their fullest potential will keep everyone on the road safer.
Finally, we asked Andreas Augsten, Team Leader Technology Strategy & Product Planning at Mobis Technical Center Europe: ‘In the context of V2X and swarm perception, what are the most promising developments that can enhance vehicle awareness and decision-making in complex driving environments?’
Andreas: By using V2X communication, swarm perception, and hybrid intelligence it is possible to improve perception and decision-making in complex driving situations.
Hybrid intelligence is combining human and artificial intelligence to improve driving decisions by leveraging human intuition and AI’s strength to process large amount of data quickly and deciding based on statistical analysis. Effective communication between human drivers and AI systems is important in this setup. New types of user interfaces are required which clearly present information and recommendations from the AI, as well as enabling humans to provide feedback or override decisions of the AI when needed. Systems enabled with hybrid intelligence can realize a learning process by integrating human feedback into the AI’s algorithms via over-the-air software updates. When a human driver intervenes in a situation with an incorrect decision by the AI, that experience can be stored and used to enhance the AI’s future performance.
Swarm perception, enabled by V2X communication, allows vehicles to collect information about their surroundings from sensors (cameras, radar, lidar, etc.) located in the road infrastructure as well as from other vehicles, creating a more complete picture of the environment. This includes detecting obstacles, traffic conditions, vulnerable road users, and hazards that are not directly visible for the sensors of the vehicle. By using the additional perception data received it is possible to improve the prediction of other traffic participants leading to safer and more informed decision-making. For example, better driving decisions in complex scenarios can be made collaboratively in case of merging lanes, at intersections, and to enhance traffic flow.
By integrating V2X, swarm perception, and hybrid intelligence, synergies can be realized leading to better situational awareness, decision-making, efficiency, and safety. Applying this approach paves the way for a more connected and intelligent transportation ecosystem.
So how important is collaboration throughout the ecosystem for navigating these challenges and successfully achieving autonomy? And why does AutoSens make a great platform for this? Bahman Hadji Senior Manager of Product Marketing – Automotive Sensing Division at onsemi, explains:
Bahman: Collaboration across the ecosystem is vital for navigating the challenges involved in solving the complex problem of autonomy. An image sensor supplier like onsemi provides the “eyes” of the system and must work in concert with a host of companies across the automotive technology ecosystem which all work to contribute to the end result desired by automotive OEMs implementing ADAS and self-driving features in passenger vehicles and fleet manufacturers providing mobility as a service.
The collaboration involves understanding the application requirements and specifications of partnering technologies’ components, often resulting in partnering on joint reference designs that outline how to manufacture an automotive-qualified camera module with optimized image quality, or implementing sensor software models for algorithm partners and SoC manufacturers which serve as the “brains” of the system to simulate the effects of different scene conditions on their autonomous driving software stack in advance of having the hardware available. Close cooperation accelerates these developments, as companies can only be successful in bringing to market their products by understanding the end application and bringing in the expertise of partners and customers to help optimize their piece in the puzzle to advance the safety and adoption of autonomous vehicles.
AutoSens is the perfect platform for these conversations to take place – it is the marquee event for key industry thought leaders, engineers, and decision makers in the space, always providing for insightful talks and stimulating discussions. The event has only grown since its inception in 2016 when the autonomous driving industry was in its infancy, and I look forward to attending again this year.
We can see from these insights that discussions at AutoSens Europe 2024 will underscore the critical role of advanced sensing technologies in shaping the future of autonomous driving and ADAS systems. From pioneering new metrological techniques to enhancing camera system performance under challenging conditions, the insights shared by industry leaders point to a safer, more reliable automotive landscape. As these innovations continue to evolve, they promise not only to enhance vehicle visibility but also to redefine the standards of safety and efficiency on the roads of tomorrow. Click here to shape these conversations with an AutoSens Europe pass.
Connect on this topic in the exhibition
AutoSens and InCabin include a technology exhibition with an array of technical demonstrations, vehicle demonstrations, buck demos from world leading companies. Engineers who come to AutoSens and InCabin have the opportunity to not only discuss the latest trends but actually get their hands on the tech and see it in action.
Ready to join the adventure and dive into the technical world of ADAS and AV technology? Our Barcelona exhibition will feature all these companies and more at the cutting edge of this technology.