Discover the crucial relationship between windscreen optical quality and camera performance with this blog from Professor Alex Braun, where he sheds light on the lack of comprehensive answers on the subject and unveils the exciting possibilities for future advancements ahead of his Windscreen Session at AutoSens this September.
Every car has a windscreen. There isn’t a continent in the whole world where windscreens (and windshields 😄) are not being produced (well, yes, Antartica…). And while the car prototypes of front-runners in autonomous driving feature cameras on top of the roof, still the de-facto standard positioning of any front-looking camera systems is right behind the windscreen, typically in front of and slightly above the rearview mirror. You’ll easily discern those from the outside by the sometimes small, mostly pretty large black-prints with a trapezoidal cut-out where the camera is looking through the glass.
So, how good does the optical quality of the windscreen have to be in that trapezoidal region such that the camera behind it is doing ok? This is not only an obviously important question, but one that is – to a scientist like me – not answered: there is no fundamental optical, metrological or material science approach that says: we understand optical quality, and this is how it influences the performance of the camera. There are many individual examples of research in academia and technology development from the industry that tackle this difficult question – and we’ll see some exciting novel results from the industry right here in the windscreen session “Improving camera optics for windshields. Introducing novel interlayer and measurement technology” – but so far, a comprehensive answer is eluding us.
IMPROVING CAMERA OPTICS FOR WINDSHIELDS. INTRODUCING NOVEL INTERLAYER AND MEASUREMENT TECHNOLOGY
- 11:40am CET
- Thursday 21st September
- MEZZANINE
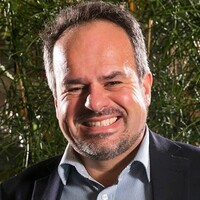
Uwe Keller
Kuraray
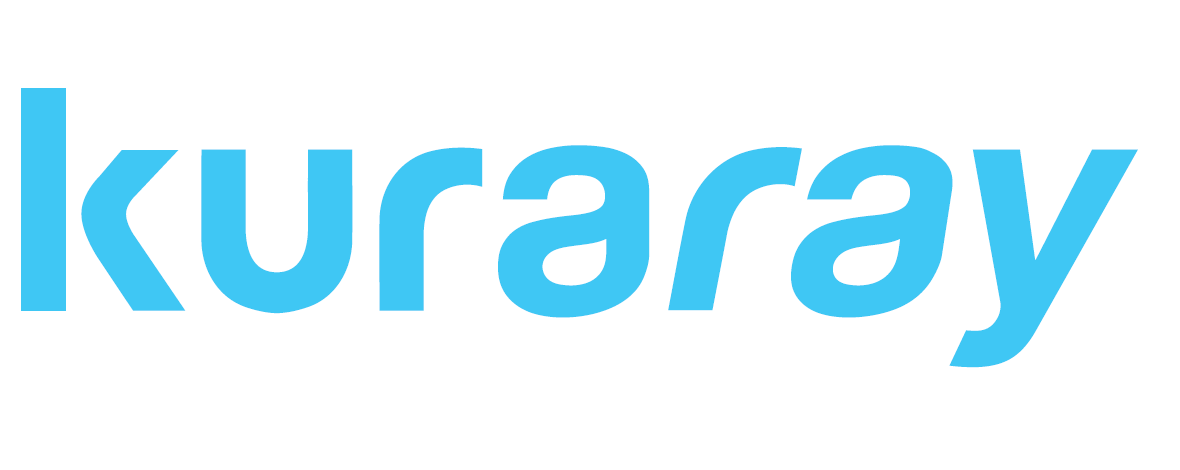
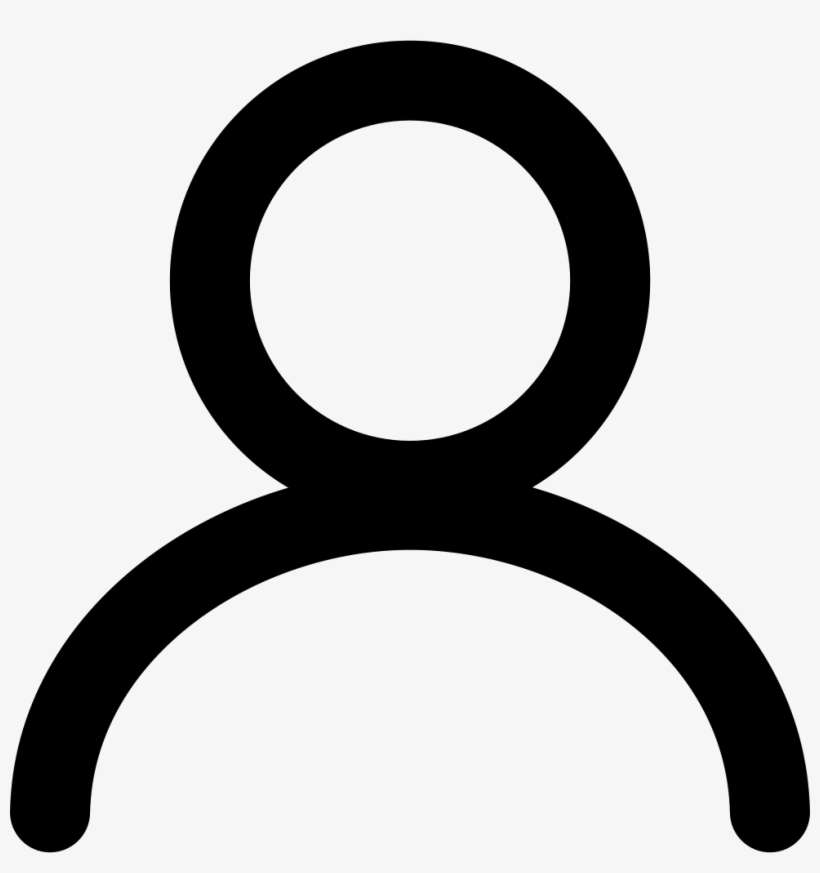
Dr. Olaf Thiele
LaVision
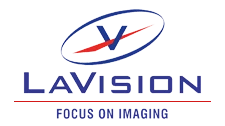
There are, of course, reasons for this. In this blog article, we’ll look at the two most important ones:
- Industry inertia (aka That’s how we’ve always done it)
- Actual harness of the problem
Industry Inertia
First and foremost, and somewhat counter-intuitively, there is an established metric on how to measure windscreens that presents a hindrance instead of a solution, the so-called refractive power. This measures the windscreen like an optometrist would do, and assigns a value like -0.25 diopters to every region of the windscreen. It has even been standardised in 1995 already! But what was this standard supposed to do? It was to say if the windscreen optical quality is good enough for a human observer to look through and not be annoyed, i.e. neither distracted nor hindered in her perception of the surrounding environment. But humans are not cameras. While a somewhat unsurprising observation, this is still not taken into account many times! We’ll dive deep into this in the session on non-biological observers.
Observing the World: Defining a New Reference Observer for Non-Biological Entities
- 4:50pm CET
- Wednesday 20th September
- MAHY
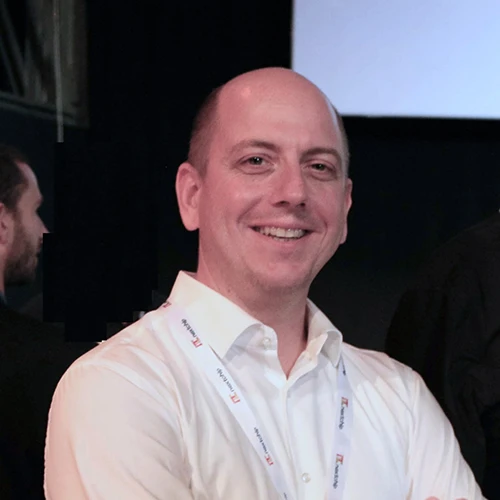
Prof. Alexander Braun
University of Applied Sciences
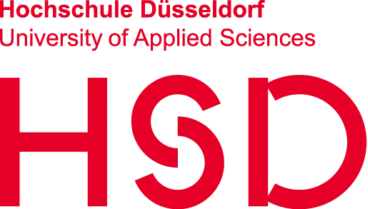

Robert Dingess
Mercer Strategic Alliance Inc.


Dr. Patrick Denny
University of Limerick
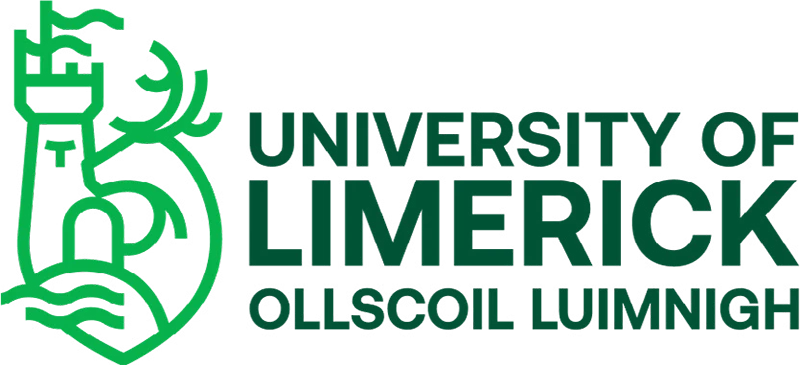
Now, when such a long time has passed in the automotive industry, and a measurement technique is so established, it won’t easily be changed, and even calling it into question is hard because of the stupendous inertia the whole process has: do we really want to change every measurement station at every windscreen manufacturer in every part of the world? Who’s gonna pay for that? Do we really have to do that? (Imagine a petulant child going “But WHY, dad??“ in a very annoying, repetitive tantrum). Unfortunately for the automotive industry and very excitingly for us we’re putting a definite pin into that!
In my talk “Windscreen Optical Quality” I’ll present the explosive results from the co-author and PhD student Dominik Wolf, from the Volkswagen Glass Laboratory (who unfortunately can’t make it himself), who mathematically demonstrates and experimentally verifies that the measurement of refractive power is fundamentally flawed, in that certain optical properties are simply not present in the measurement. But these properties have a proven influence on camera performance! Whew! Hope you want to hear more about that!
windscreen optical quality
- 12:05pm CET
- Thursday 21st September
- Mezzanine
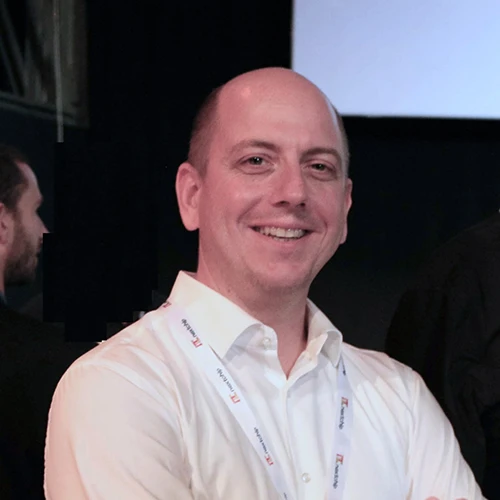
Prof. Alexander Braun,
University of Applied Sciences, Hochschule Düsseldorf
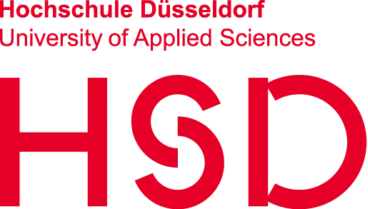
Actual Hardness of the Problem
The second and also very relatable reason why currently there isn’t a good answer to “How good does the windscreen have to be?” it’s simple: it’s a really, really hard problem! Modern camera system obtains their performance by leveraging state-of-the-art machine learning (ML) algorithms, for basically all functionality like object detection (pedestrians, cars etc.), semantic, instance or panoptic segmentation, traffic light detection, traffic sign recognition, lane detection, even optical flow — the list goes on.
These topics will be touched on throughout the agenda, with sessions focusing on camera technology, thermal imaging, v2x and more.
Why is using state-of-the-art machine learning algorithms a problem?
Because the performance of these algorithms cannot be fundamentally ascertained! It can, of course, be quantified, but due to the black-box nature of these algorithms, they may break at unexpected turns. And we apparently need a performance limit of the camera function(s) to determine a production tolerance limit for the windscreen quality. Otherwise, the windscreen quality might accidentally break the ML algorithm, which for obvious reasons, can pose a serious security challenge.
I hope I have vetted your appetite for a topic that, at first glance, might seem a bit underwhelming. And while I’m at it, please let me also highlight that you can dive even deeper into the question of linking optical quality to ML performance by coming to my tutorial on “Spatial recall index – where is the performance?”
If you know a bit about optics, you’ll appreciate that the optical quality of a camera is not constant over the whole image; quite the contrary. A typical automotive (front-looking) camera is really sharp in the middle of the image, but less so towards the edges of the image. Does a camera then detect pedestrians worse in the corner of the image, or doesn’t it matter, because the differences are too subtle and the networks generalise well enough? How would we even measure that? This is where our Spatial Recall Index (and it’s new sibling, the Generalised Spatial Recall Index, fresh out of publication) comes into play: we can now evaluate the performance pixel by pixel, and thus have a go at the above questions.
In any case, whichever sessions you choose: do not miss the AutoSens in Brussels, and I hope to see you there!