AutoSens 2023 provides new insights on thermal imaging, radar, sensor fusion, and much more. Wilfried Philips, expert at Imec and member of the AutoSens Advisory board, takes us through the key discussion points and latest research that will be featuring on the agenda.
The upcoming Autosens event features many important topics, as it does every year. As Sensor Fusion research leader within imec, my opinion on the most pertinent AutoSens topics is colored by ongoing imec research, including my own.
Three remarkable trends caught my attention:
• thermal imaging is on the rise, with emergency braking as an important use case, driven by the EU NCAP regulations;
• radar systems increasingly rely on powerful sensor fusion;
• companies are increasingly aware of the processing complexity of automotive sensing solutions, translating into novel hardware and algorithmic approaches.
Thermal Imaging and Pedestrian Emergency Braking
Despite progress in recent years, “Pedestrian Automatic Emergency Braking Systems” are still not good enough: Many fatal accidents occur at night, and this is exactly when they underperform.
Enter EU regulation No 131, which now makes “advanced emergency braking” systems mandatory. It seems that the National Highway Traffic Safety Administration is considering similar steps. While such systems can save many lives, when badly designed, they can also cause rear-end collisions. Having them operate correctly under a wide variety of weather and light conditions is far from trivial.
Improvements in camera technology, e.g. high dynamic range cameras, have made visual analytics gradually more reliable, but such solutions still require a minimal level of light. LiDaR is still quite expensive. As confirmed by our own research, radar is a very promising and low-cost sensor, with even higher when fused with an imager.
Thermal cameras show great promise as they require no light at all. Instead, they detect humans and animals by their body heat. However, the state of the art in thermal visual analytics and in thermal imaging sensor fusion lags that of “regular” cameras. Thermal cameras are used in traffic management, surveillance and remote sensing and military applications. However, thermal image analysis is quite different from RGB image analysis: thermal pictures tend to have lower resolution, lack color, and look very different from reality. Some people even complain that thermal pictures makes them look older. Thermal image analysis is also confounded by outside temperature variation: humans may appear darker or brighter than the background, or – at the right temperature – become invisible.
In summary, thermal cameras show great promise, but require more research. AutoSens will feature presentations on improving optics of thermal cameras, on emergency braking applications and even on distance estimation from thermal images using AI. Check out the agenda here.
THERMAL IMAGING AND PAEB Q&A PANEL
- 5:10pm CET
- Wednesday 20th September
- Mezzanine Stage

Bendix De Meulemeester
Director Marketing & Business Development
Umicore
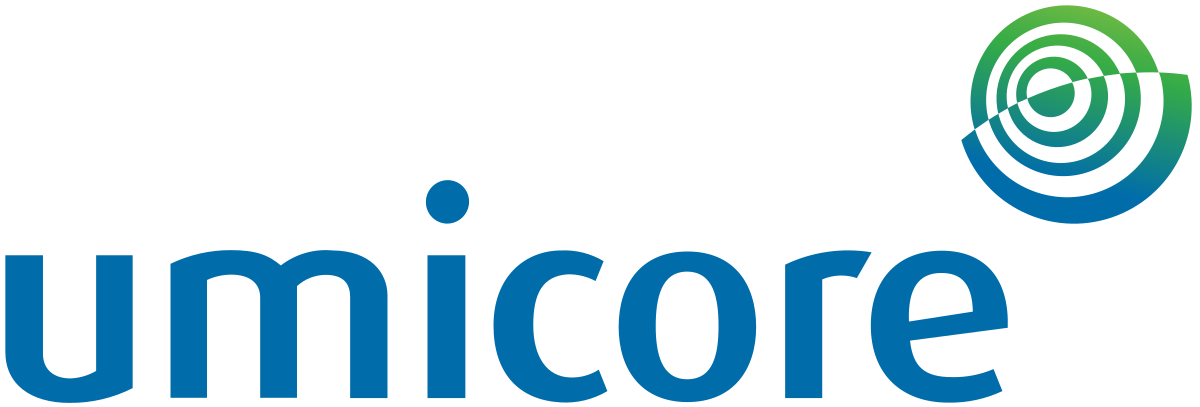
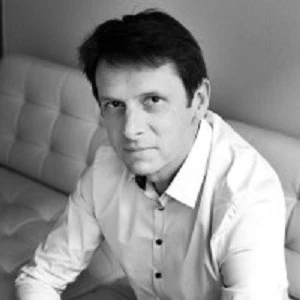
Sébastien Tinnes
Global Market Leader
Lynred
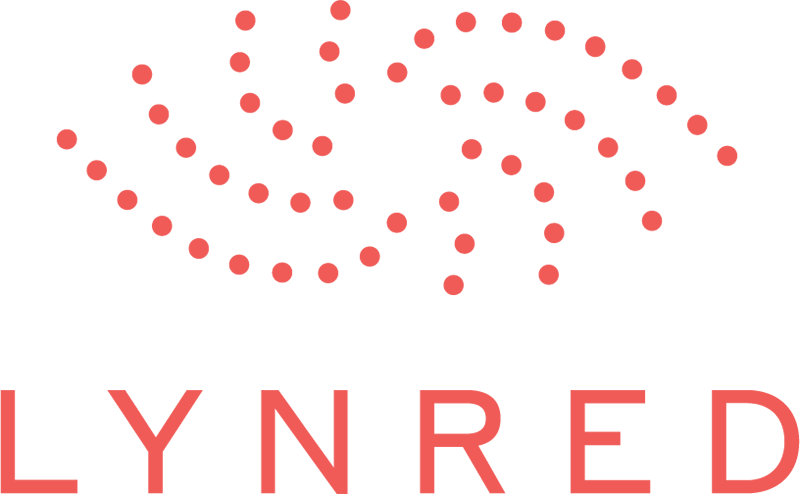
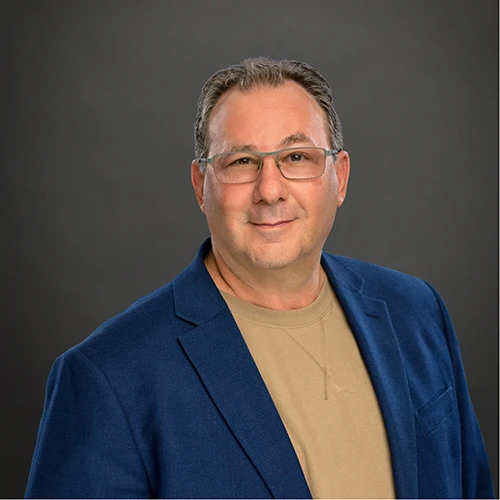
Chuck Gershman
CEO, Co-Founder
OWL AI
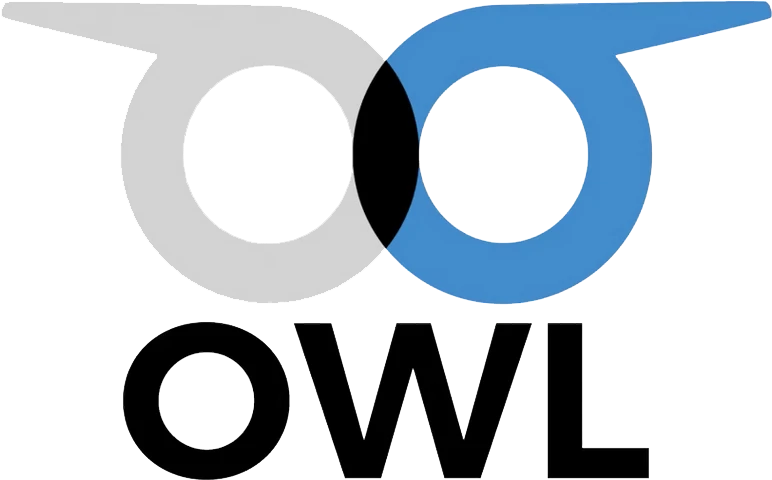
Unlocking the potential of radar
Cameras currently provide the highest detail on objects in the scene, under favorable light conditions. They can detect road users at large distances, distinguish between people and animals, pedestrians and cyclist… But they have their limits, especially in poor weather (rain or fog). Moreover, they lack direct depth perception. Radar does provide direct depths clues, and therefore naturally complements cameras. It is also the sensor type least likely to fail in adverse weather conditions.
One of the downsides of radar is its comparatively low angular resolution, which is a consequence of the physics of radio waves. As its name suggests, mm-wave Radar employs radio wavelengths much larger than those of (infrared) light: millimeters rather than micrometers. This limits the directional resolution of Radar, as the Radar beam width decreases with wavelength. However, this can also be an advantage: distant traffic poles missed by LiDaR because they happen to be just missed by the LiDaR beams will still produce strong reflections of the much “broader” radar beam.
Radar resolution can be improved by moving to shorter wavelengths (e.g., imec’s 144Ghz radar) or by combining multiple radars physically separated on the of car. Such a solution effectively replaces the “small” radar with a much larger virtual antenna, which improves angular resolution. Such multi-radar solutions do require very accurate time synchronization, adding complexity, but this is not insurmountable.
Modern automotive radars use electronic beam steering to scan the traffic scene. Imaging radar relies on narrow radar beams and advanced beam steering, combining many antennas. Despite such advances, the resulting “distance” images still have relatively low angular resolution compared to LiDaR. A promising approach is therefore to use radar and camera images, with the radar providing precise distance resolution and the camera adding angular resolution and color.
In summary, radar provides ever higher native resolution, which can be enhanced even more by camera sensor fusion. AutoSens will feature presentations on this “super resolution” approach allowing all-weather perception, on neural networks for implementing such superresolved distance images, but also for object detection, classification and free-space estimation. Another interesting topic is sensor and signal processing parameter adaptation to better sense specific traffic scenes. Click here to see what’s on the agenda.
FROM VISION TO ACTION: ELEVATING SAFETY WITH SUPER-RESOLUTION IMAGING RADARS AND AI-ENABLED PERCEPTION
- 12:40pm CET
- Wednesday 20th September
- Minerva Room
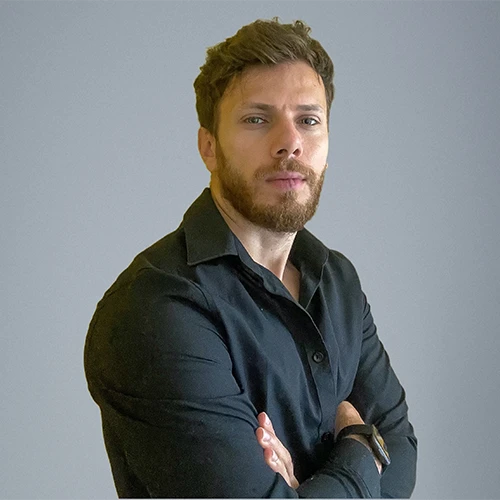
Dane Mitrev
Senior ML Engineer
Provizio ai
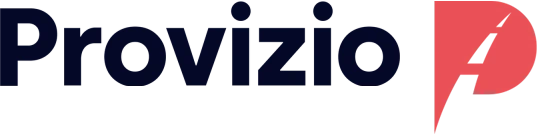
SOFTWARE-DEFINED RADAR SENSORS FOR AUTONOMY CUSTOMERS
- 4:10pm CET
- Wednesday 20th September
- Minerva Room

Dr. Ralph Mende
CEO and Founder
smartmicro
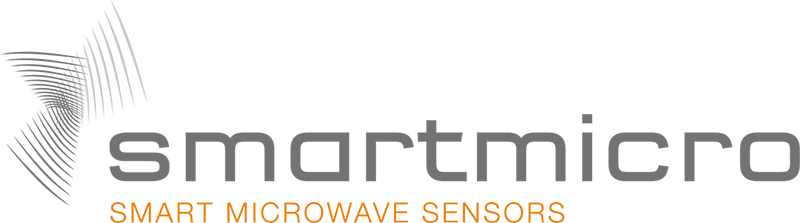
REDEFINING RADAR – CAMERA SENSOR FUSION: A LEAP TOWARDS AUTONOMOUS DRIVING WITHOUT LIDAR
- 11:15am CET
- Thursday 21st September
- Mahy Room
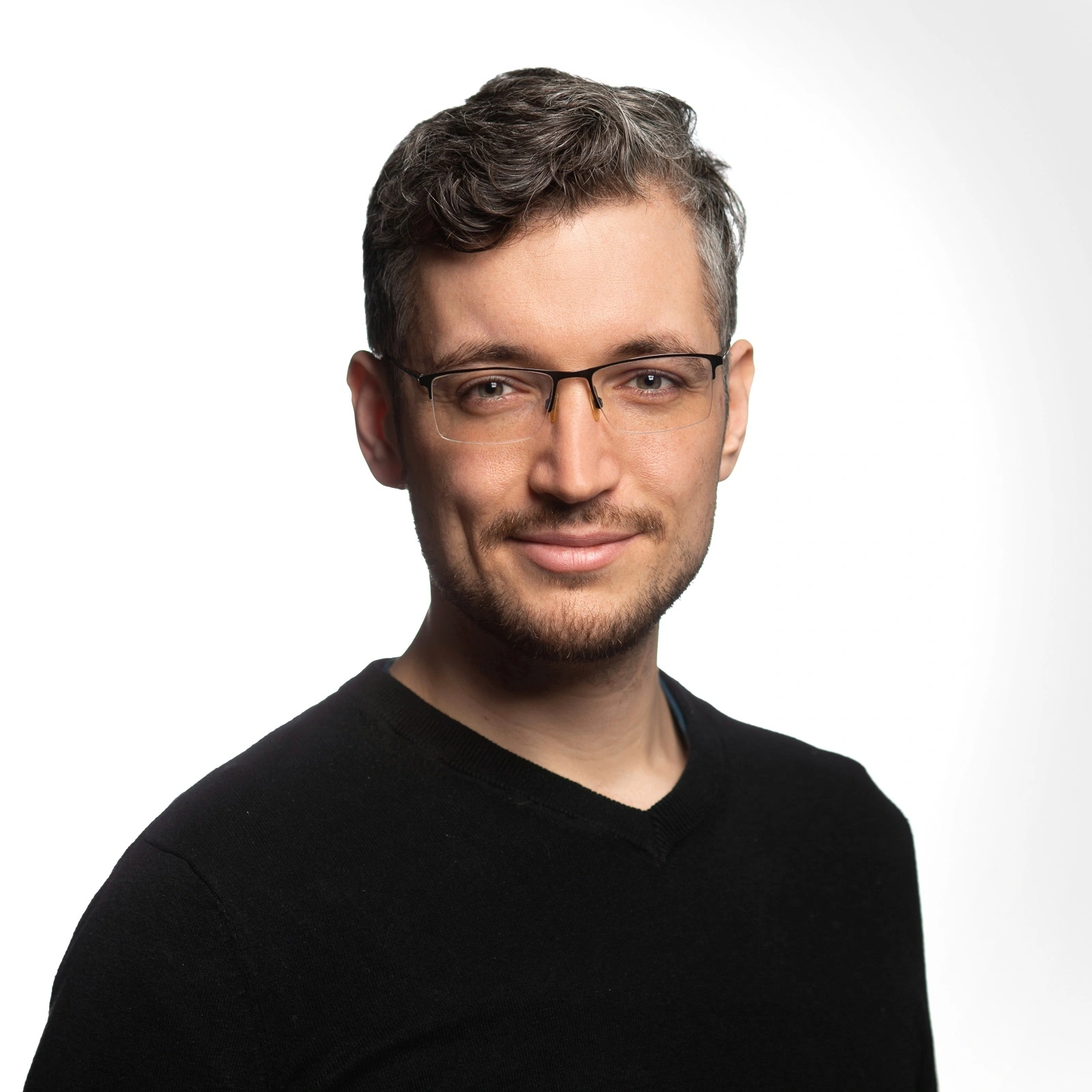
Andras Palffy
Co-Founder
Perciv AI

Sensor fusion architectures for autonomous driving
Autonomous driving is still in its infancy, and has not even reached the consumer market. In order to do so, it must be made safer and more reliable and far less expensive. This will require many sensors on the car, producing massive amounts of data. The cost of these sensors, but also of processing the massive amounts of data must be greatly reduced.
In the community, the debate on the relative merits of centralized, zonal and decentralized compute architectures has not been settled. In the near future, a centralized processing and fusion architecture seem to fit best with some important OEM and TIER-1 roadmaps. However, some of the best current stand-alone “smart” sensors rely on embedded processing, taking advantage of easier certification, faster reaction times and higher reliability.
Centralized architectures are attractive because the separation of compute and sensing resources offers more choice in selecting sensors, more flexibility in reallocating computational and memory resources between sensors. A single compute platform also avoids the potential problems of needing to support different sensor specific platforms.
However, centralized compute platforms have several important downsides that will render them less and less attractive when levels of automation increase. They constitute single points of failure and will need to be duplicated as backup systems, just as essential sensors and control systems in airplanes are. They concentrate all heat production in a single box, limiting the number of computations. They rely on high-speed links, adding to cost. The zonal architecture is a better compromise in this respect.
My personal prediction is that architectures will become more and more decentralized over time, but before this happens, consensus will need to grow on what types of data to exchange between sensors and compute units, and in what level of detail. Irrespective of the chosen architecture, the energy demands and the cost of solutions built with current hardware and software need to be improved by a large factor for consumer autonomous driving to be realistic at all.
The cheapest computations are those that are never made at all. Active sensing strategies can therefore be part of the solution: carefully adapting frame rates, processing only well-chosen parts of the data or – even better – not sensing all parts of the scene with all sensors all the time. Perhaps this too will be addressed at a future AutoSens.
Meanwhile, one way to reduce computations is context-adaptive processing: instead of relying on large neural networks handling all possible combinations of scenes, weather and light conditions… we can use a smaller network, designed only to handle standard weather and light conditions. The clever bit is to add a smaller auxiliary neural network to adapt the main network’s output to compensate for weather and light influences. This calibrates the “almost final” output of the neural network for the current sensing conditions. This not only saves computation, but also requires less training.
Improvements in neural networks (e.g., power driven architecture search) are another part of the solution. On the hardware level, novel processing paradigms, e.g., neuromorphic neural networks promise lower power consumption and ultrafast response times at the lowest levels of processing.
No matter what processing hardware is selected, neural networks require massive amounts of information transfer, internally in high-end compute chips. Chiplets and “networks on chips” promise to provide power-efficient, massively parallel internal data transfer. Chiplets also enable a new, modular approach to customizing high-end Application Specific Integrated Circuits to specific automotive needs. This solves another major problem: the prohibitively expensive Non Recurring Engineering cost of their design.
In summary, the data processing challenges of automotive sensor fusion will be addressed at the hardware and software level. AutoSens features presentations and a panel discussion on future hardware processing architectures and on design methodologies for low power neural networks. Other presentations will address new fusion methods to avoid needless computations, thus providing complementary solutions.
HOW DEVELOPMENTS RELATED TO AUTOMATED DRIVING ARE INFLUENCING THE SENSOR AND COMPUTING MARKETS
- 11:55am CET
- Wednesday 20th September
- Mahy Room

Pierrick Boulay
Senior Analyst – Lighting and ADAS systems
Yole Group
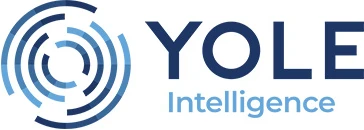
CONTEXT ADAPTATION FOR AUTOMOTIVE SENSOR FUSION
- 11:40am CET
- Thursday 21st September
- Mahy Room

Jan Aelterman
Assistant Professor
Gent University
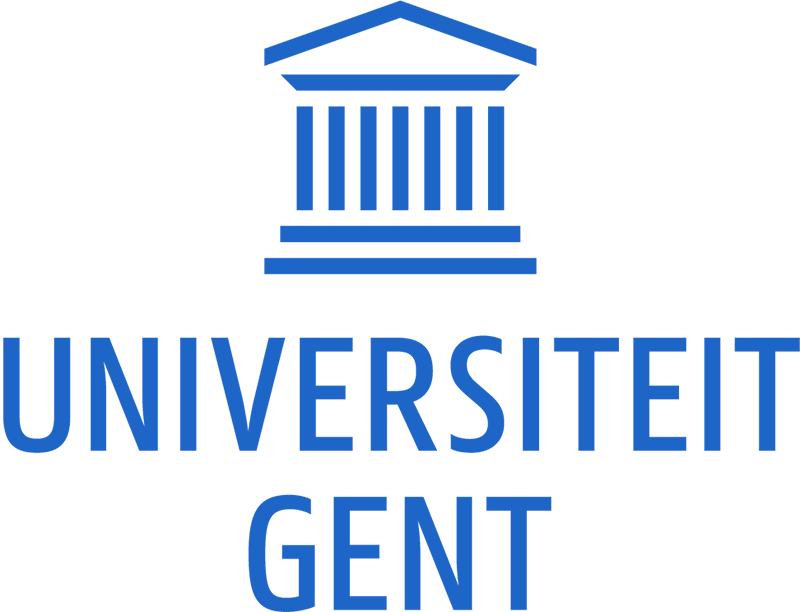
NETWORK-ON-CHIP DESIGN FOR THE FUTURE OF ADAS AI/ML SEMICONDUCTOR DEVICES
- 11:40am CET
- Thursday 21st September
- Minerva Room
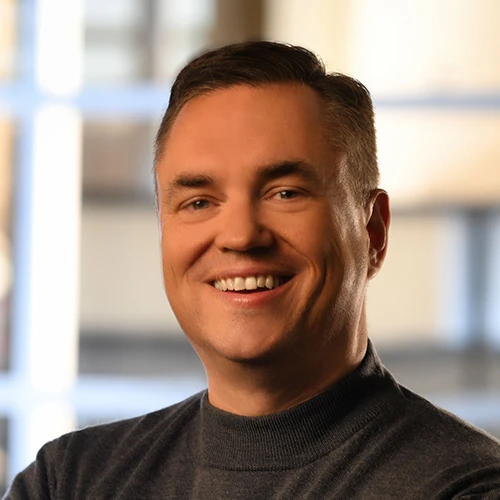
Frank Schirrmeister
Vice President of Solutions and Business Development
Arteris
